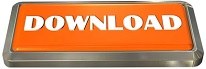

Something historical- Executive Order 9066 authorized the internment of Japanese Americans in 1942. With all that in mind, we identified a few sample documents to run through OCR systems so we could compare the results:Ī receipt-This receipt from the Riker’s commissary was included in States of Incarceration, a collaborative storytelling project and traveling exhibition about incarceration in America.Ī heavily redacted document- Carter Page’s FISA warrant is a legal filing with a lot of redacted portions, just the kind of exasperating thing reporters deal with all the time. DocUNet: Document Image Unwarping via a Stacked U-Net is a good introduction to scholarly theory on image pre-processing.
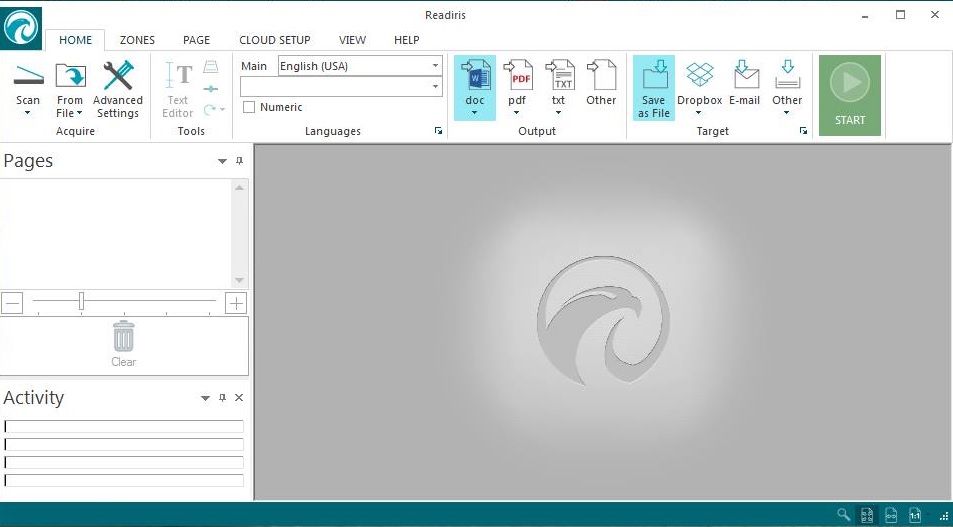
If you’re interested in going deep on the future of OCR, Scene Text Detection and Recognition: The Deep Learning Era is an excellent survey of current literature on scene recognition. There is also a good deal of promising research on techniques for pre-processing images-doing things like straightening out warped text, super resolution to boost missing details, spotting text in arbitrary locations or at odd angles, and techniques for accommodating lower resolution text. Scene recognition engines have to be better about spotting letter glyphs. Current OCR tools often choke on font changes, inline graphics, and skewed text-scene recognition has to accommodate all of those hurdles.
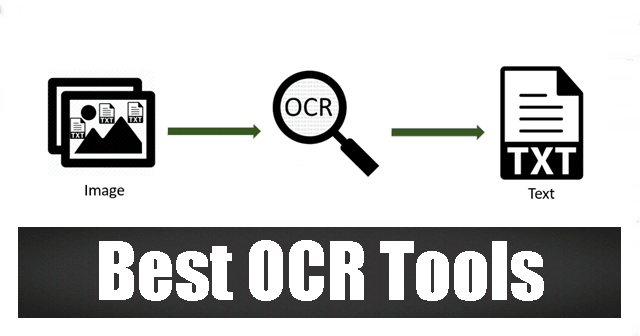
As researchers and programmers look for ways to identify text in the wild (think street signs and package labels) and not just on linear documents, they’re developing tools that do a better job of identifying and interpreting text that isn’t neatly arranged in rows and paragraphs. The most promising advances in OCR technology are happening in the field of scene text recognition. A dictionary isn’t always enough, however, as Wesley Raabe learned as he was transcribing the 1879 edition of Uncle Tom’s Cabin. Some use a dictionary to improve results-when a string is ambiguous, the engine will err on the side of the known word. Most start with a line detection process that identifies lines of text in a document and then breaks them down into words or letter forms. They assume that material fits on a rectangular page. The current slate of good document recognition OCR engines use a mix of techniques to read text from images, but they are all optimized for documents. In most cases if you need a complete, accurate transcription you’ll have to do additional review and correction. None got perfect results on trickier documents, but most were good enough to make text significantly more comprehensible. Most of the tools handled a clean document just fine. The quality of results varied between applications, but there wasn’t a stand out winner. You can use the scripts to check our work, or to run your own documents against any of the clients we tested.
#BEST OCR TOOL FREE#
We tested three free and open source options (Calamari, OCRopus and Tesseract) as well as one desktop app (Adobe Acrobat Pro) and three cloud services (Abbyy Cloud, Google Cloud Vision, and Microsoft Azure Computer Vision).Īll the scripts we used, as well as the complete output from each OCR engine, are available on GitHub. We selected several documents-two easy to read reports, a receipt, an historical document, a legal filing with a lot of redaction, a filled in disclosure form, and a water damaged page-to run through the OCR engines we are most interested in.

Some are quite expensive, some are free and open source. Some are easy to use, some require a bit of programming to make them work, some require a lot of programming. There are a lot of OCR options available. We couldn’t find single side by side comparison of the most accessible OCR options, so we ran a handful of documents through seven different tools, and compared the results. We have been testing the components that already exist so we can prioritize our own efforts. One of our projects at Factful is to build tools that make state of the art machine learning and artificial intelligence accessible to investigative reporters. OCR, or optical character recognition, allows us to transform a scan or photograph of a letter or court filing into searchable, sortable text that we can analyze. Do you need to pay a lot of money to get reliable OCR results? Is Google Cloud Vision actually better than Tesseract? Are any cutting edge neural network-based OCR engines worth the time investment of getting them set up?
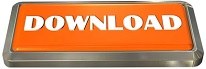